Hiện tại, Cakhia TV đang nổi lên như là một trang web trực tiếp bóng đá được yêu thích hàng đầu Việt Nam và khu vực. Bởi dù chỉ mới xuất hiện cách đây không lâu, chúng tôi đã đáp ứng số lượng người truy cập website xem bóng đá trực tuyến mỗi ngày vô cùng lớn.
- Cakhia TV là gì?
- Mục tiêu phát triển của kênh trực tiếp bóng đá Cakhia TV
- Kênh bóng đá trực tuyến hàng đầu khu vực hiện nay – Cakhia TV
- Trang web truc tiep bong da Cà Khịa TV có gì nổi bật?
- Những lưu ý khi xem truc tiep bong da tại Cakhia TV
- Những lý do mà bạn nên xem tructiepbongda tại Cakhia TV
- Tổng hợp một vài website xem trực tiếp bóng đá khác
Cakhia TV là gì?
Cakhia TV hiện đang là phát sóng trực tiếp bóng đá hàng đầu Việt Nam bởi chất lượng đường truyền ổn định, dàn bình luận viên Giàng A Phò, Giàng A Lử sẵn sàng cháy bùng nhiệt sôi động cùng fan hâm mộ mỗi khi có nhu cầu xem bóng đá trực tuyến tốc độ cao.
Khi nhận thấy được nhu cầu xem tructiepbongda tại Việt Nam tăng khá nhanh. Tuy nhiên các website hiện tại vẫn chưa đủ điều kiện để đáp ứng. Ngay lập tức trang web Cà Khịa TV được ra đời với việc đứng sau là những biên tập viên, chuyên gia bóng đá hàng đầu.

Thêm vào đó, chúng tôi còn đầu tư khá nhiều tiền để xây dựng một website xem trực tiếp bóng đá hoàn thiện nhất. Cho nên chỉ trong một thời gian ngắn, Cakhia TV đã xây dựng được thương hiệu của mình. Khi là sự lựa chọn của rất nhiều người, nếu muốn xem bóng đá trực tiếp. Bằng chứng là mỗi ngày, chúng tôi đón nhận hàng chục nghìn lượt truy cập.
Khi vào Cà Khịa TV, bạn sẽ thấy toàn bộ các giải bóng đá lớn nhất trên thế giới và trong nước đều được phát sóng đầy đủ. Giúp bạn thưởng thức được các trận đấu đỉnh cao mỗi ngày khá là dễ dàng mà lại hoàn toàn miễn phí.
Chúng tôi còn ứng dụng cả những công nghệ tructiepbongda hiện đại nhất. Cho nên các trận đấu được phát sóng tại Cakhia TV luôn có chất lượng cực kỳ cao. Trang web CaKhia Live còn sử dụng cả đường truyền P2P, nên tốc độ trận đấu diễn ra khá là mượt mà và ổn định.
Đặc biệt nhất, Cà Khịa Live còn có một đội ngũ BLV khá là chuyên nghiệp và đông đảo. Với những cái tên cực kỳ quen thuộc với những anh em thường xuyên xem bong da truc tiep. Để tham gia bình luận vào các trận đấu lớn, giúp không khí xem bóng đá của bạn trở nên sinh động hơn rất nhiều.
Mục tiêu phát triển của kênh trực tiếp bóng đá Cakhia TV
Ngay từ ngày đầu xây dựng, chúng tôi đã đặt mục tiêu trở thành website truc tiep bong da hàng đầu Việt Nam. Cho nên đã đầu tư rất nhiều tiền và công sức, để xây dựng một trang web hoàn chỉnh nhất. Để cung cấp cho mọi người những trận đấu bóng đá hấp dẫn và chất lượng.
Thời điểm hiện tại, dù chỉ mới được xây dựng không quá lâu nhưng Cà Khịa Live đã có những thành tựu ban đầu. Khi mà địa điểm quen thuộc của rất nhiều người, khi có nhu cầu xem các trận đấu bóng đá mình thích trên điện thoại hay máy tính.
Đương nhiên đây chỉ là những bước ban đầu, nhưng với sự đầu tư, cố gắng của đội ngũ Ca Khia TV. Chắc chắn trong tương lai gần, chúng tôi sẽ trở thành một website xem tructiepbongda hàng đầu Việt Nam. Một nơi mà tất cả những anh em thích xem bong da truc tiep đều biết đến.
Kênh bóng đá trực tuyến hàng đầu khu vực hiện nay – Cakhia TV
Theo như khảo sát, đa số mọi người từng xem tructiepbongda tại CaKhia Live đều cực kỳ hài lòng với những gì mà chúng tôi mang lại. Bởi tại đây, mọi người không chỉ được xem trực tiếp trận đấu bóng đá mình thích với chất lượng cao và trải nghiệm tốt. Mà còn được sử dụng thêm nhiều các chức năng bổ ích khác. Cụ thể đó là:
- Tin tức: Chúng tôi mỗi ngày đều tìm kiếm và cập nhật toàn bộ các thông tin bóng đá mới nhất trong nước cũng như thế giới. Để bạn biết được tin tức về cầu thủ, huấn luyện viên, trước trận đấu, giải đấu, chuyển nhượng,.. hot nhất trong 24 giờ qua
- Lịch thi đấu: Trang web Cà Khịa TV hiện tại cũng đang cập nhật lịch thi đấu cho toàn bộ các giải bóng đá trên thế giới và trong nước. Nên bạn có thể biết được lịch của những trận đấu hấp dẫn hay các đội bóng mà mình thích dễ dàng
- Bảng xếp hạng: Tại đây, bạn sẽ xem được bảng xếp hạng ở bất kỳ giải đấu bóng đá nào mà mình muốn. Để biết thành tích các đội bóng trên bảng xếp hạng hay là đội bóng mà mình yêu thích chuẩn nhất

- Kết quả: Chúng tôi cũng cập nhật kết quả toàn bộ các trận đấu bóng đá vừa mới diễn ra. Để bạn biết được tỷ số những trận đấu mà mình không thể xem trực tiếp như thế nào chuẩn nhất
- Highlight: Đây là chức năng giúp bạn xem lại được video highlight các trận đấu bóng đá lớn vừa mới khép lại. Để biết diễn biến chính của trận đấu như thế nào, cầu thủ ghi bàn ra sao, các tình huống hấp dẫn nhất,… trong thời gian khá ngắn
- Tỷ lệ kèo: Bạn sẽ xem được bảng kèo toàn bộ các trận đấu bóng đá chuẩn bị diễn ra với đầy đủ thông tin cực kỳ chuẩn. Giúp bạn soi kèo bất kỳ trận đấu bóng đá nào mà mình muốn dễ dàng hơn
- Tips: Đây là chức năng giúp bạn tham khảo được những kinh nghiệm soi kèo, mẹo cá cược bóng đá hay từ những cao thủ hàng đầu. Để giúp bạn có thể tăng tỷ lệ thắng của mình khi tham gia cá cược bóng đá
- Livescore: Đây là ứng dụng mà chúng tôi cung cấp, để bạn xem tỷ số trực tiếp các trận đấu đang diễn ra. Giúp bạn biết kết quả những trận đấu mà mình không thể xem tructiepbongda được.
Cũng như xem bong da truc tiep, bạn có thể truy cập vào Cà Khịa Live để sử dụng các chức năng trên đây bất kỳ lúc nào mà không cần phải trả phí.
Trang web truc tiep bong da Cà Khịa TV có gì nổi bật?
Không phải ngẫu nhiên mà chúng tôi lại được rất nhiều người xem đánh giá cao và truy cập vào để xem trực tiếp bóng đá mỗi ngày. Mà bởi vì Cà Khịa TV có những ưu điểm vượt trội sau đây:
Giao diện website Cakhia đẹp mắt
Trước khi xây dựng website của mình, chúng tôi đã khảo sát hành vi người dùng khá là nhiều. Để thiết kế ra một trang web có giao diện cực kỳ dễ sử dụng. Mà ngay cả những người không biết quá nhiều về công nghệ cũng có thể sử dụng dễ dàng.
Thêm vào đó, màu mắt mà chúng tôi lựa chọn cũng khá hài hòa. Để làm nổi bậc các chức năng quan trọng. Để bạn tìm những thông tin mà mình cần nhanh nhất. Cũng như không bao giờ cảm thấy bị đau mắt, nếu nhìn quá lâu vào màn hình.

Tại chuyên mục Xem Bóng Đá Trực Tiếp, chúng tôi còn chia ra nhiều mục như giải đấu, các trận đấu diễn ra vào hôm nay, ngày mai, trận đấu hot nhất,… Giúp bạn dễ dàng tìm được trận đấu bóng đá mà mình muốn xem.
Một điểm cộng nữa mà CaKhia TV không chèn quá nhiều banner quảng cáo ở trang chủ. Nên bạn sẽ không bị làm phiền, khi truy cập vào đây. Trang web CaKhia Live còn sử dụng đường truyền khá hiện đại, nên tốc độ load website cũng khá nhanh.
Đặc biệt, Cakhia TV được xây dựng để tương thích với toàn bộ các hệ điều hành, từ IOS, Window Phone, Android. Để bạn truy cập vào đây bằng bất kỳ thiết bị nào, từ máy tính, điện thoại, laptop,.. đều có thể sử dụng mượt mà.
Phát sóng đa dạng giải đấu
Khi truy cập vào Cà Khịa Live để xem truc tiep bong da, bạn cũng thấy gần như toàn bộ các giải đấu hấp dẫn từ trong cho đến ngoài nước đều được phát sóng đầy đủ. Trong đó những giải đấu hấp dẫn nhất phải kể đến như:
- Giải vô địch Ngoại hạng Anh – Premier League
- Cúp C1 – UEFA Champions League
- Giải vô địch bóng đá Tây Ban Nha – La Liga
- Giải đấu bóng đá Ý – Serie A
- Giải bóng đá vô địch quốc gia Đức – Bundesliga
- Giải đấu quốc gia Việt Nam – V-League
- Các giải đấu mà đội tuyển Việt Nam tham dự
- Ngoài ra còn rất nhiều các giải bóng đá khác tại Châu Á, Châu Âu, Chây Mỹ,…
Với việc phát sóng toàn bộ các giải đấu hàng đầu trong nước và cả thế giới hiện nay. Chỉ cần bạn vào Cakhia Live là có thể xem tructiepbongda bất kỳ trận đấu nào một cách dễ dàng.
Trang web Cà Khịa TV còn cập nhật cả lịch phát sóng bóng đá trực tiếp vào đầu giờ mỗi ngày với đầy đủ thông tin. Để bạn biết được hôm nay diễn ra các trận đấu nào, thời gian thi đấu ra sao,.. Để bạn thu xếp thời gian hợp lý để xem trực tiếp trận đấu bóng đá mình thích.
Xem full HD tại Cakhia5 TV
Chúng tôi là một trong những website phát sóng bong da truc tiep đầu tiên tại Việt Nam áp dụng những công nghệ 4.0. Cho nên khi xem trực tiếp bóng đá tại đây, bạn sẽ cảm nhận được chất lượng cực kỳ cao mà ít nơi nào có được. Cụ thể như sau:
- Trận đấu luôn có độ phân giải hình ảnh Full HD, giúp bạn cảm nhận được sự sắc nét về hình ảnh
- Âm thanh của trong trận đấu khá chân thực đi kèm với âm lượng lớn nên sống động hơn rất nhiều, giúp bạn cảm nhận được sự sôi động trên khán đài
- Kích thước màn hình mà chúng tôi cung cấp cũng đúng chuẩn và tương thích với tất cả mọi thiết bị
Ngoài ra, Ca Khia TV còn cho phép bạn thay đổi kích thước màn hình, tăng giảm âm lượng hay độ phân giải hình ảnh. Sao cho bạn có thể xem trận đấu bóng đá mà mình muốn một cách tốt nhất.
Xem với tốc độ cao
Một điểm mạnh nữa của chúng tôi, đó chính là sử dụng đường truyền với công nghệ hiện đại. Cho nên khi bạn xem tructiepbongda tại đây. Bạn sẽ cảm nhận được tốc độ mà trận đấu diễn ra cực kỳ ổn định, mượt mà. Không khác gì bạn xem trên TV cả.
Các hiện tượng gây khó chịu cho người xem như giật, lag hay là đứng hình cũng gần như rất ít khi xuất hiện, nếu bạn có kết nối mạng tốt. Còn khi xuất hiện, bạn chỉ cần dừng trận đấu lại khoản vài giây là có thể tiếp tục xem mà không bao giờ gặp lại nữa.
Đội ngũ bình luận viên chuyên nghiệp, máu lửa
Đây chính là ưu điểm được rất nhiều người đánh giá cao tại trang web CaKhia TV. Khi hiện tại, chúng tôi đang có trong đội ngũ rất nhiều BLV tên tuổi tại Việt Nam. Để tham gia bình luận các trận đấu bóng đá hấp dẫn nhất mỗi ngày. Giúp bạn xem bong da truc tiep với trải nghiệm cực kỳ cao.
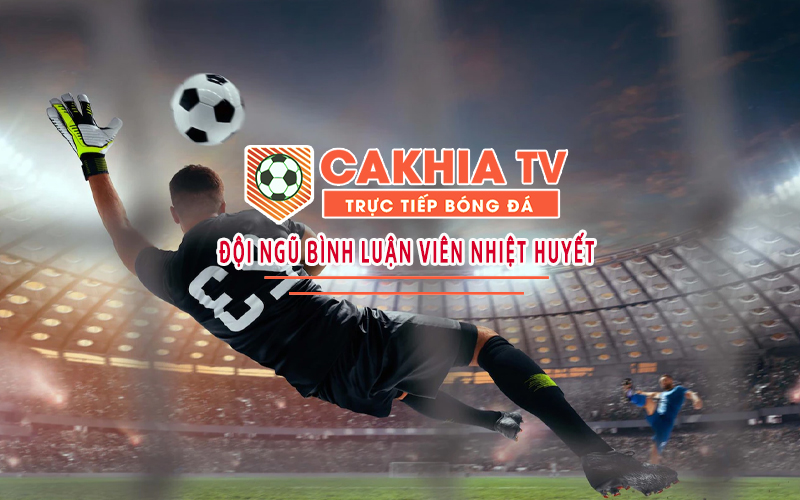
Khi xem trực tiếp bóng đá tại đây, bạn cũng sẽ thấy các BLV trong trận đấu luôn luôn tương tác với người xem rất nhiều. Điều này sẽ tạo một không khí cực kỳ sinh động, mà đảm bảo rằng bạn sẽ có những giây phút xem bóng đá vui vẻ nhất.
Ngoài ra, bạn cũng có thể giao lưu với các bình luận viên trong trận đấu khá dễ dàng. Chỉ cần bạn đặt những câu hỏi về bóng đá, cá cược hay là thể thao. Cho những BLV này trả lời, giúp bạn không cảm thấy buồn chán khi xem bóng đá một mình.
Hệ thống link chất lượng
Trang web truc tiep bong da CaKhia TV hiện tại cũng cung cấp đường link xem trực tiếp các trận đấu bóng đá cực kỳ chất lượng. Để giúp bạn tìm được đường link trong trận đấu mình muốn để dàng. Cũng như theo dõi trận đấu một cách trọn vẹn hơn. Cụ thể như sau:
- Hiện tại thì chúng tôi cập nhật link xem tructiepbongda hoàn toàn miễn phí. Nên bạn có thể vào đây để lấy link xem trận đấu mình thích bất kỳ lúc nào
- Thời gian mà CaKhia Live cập nhật link xem bong da truc tiep cũng khá sớm, cụ thể là trước khi trận đấu bắt đầu 1 tiếng. Để bạn lấy được link xem bóng đá sớm nhất
- Mỗi trận đấu bóng đá được phát sóng tại Cakhia TV cũng được cập nhật trên 3 đường link cho bạn lựa chọn. Cũng như nếu link bạn đang xem gặp bất kỳ vấn đề gì, bạn có thể chuyển sang link khác ngay lập tức
- Trang web Cà Khịa TV cũng cam kết với mọi người rằng các đường link xem trực tiếp bóng đá được cập nhật tại đây cũng không bao giờ dính virus hay là mã độc. Cho nên bạn hoàn toàn có thể yên tâm.
Không chèn quảng cáo
Một lợi ích nữa khi bạn xem tructiepbongda tại CaKhia TV, đó là gần như rất ít khi nào thấy quảng cáo, banner hay popup được chèn vào trong trận đấu. Bởi ngay từ những ngày đầu phát triển và xây dựng, chúng tôi đã muốn trải nghiệm của người xem là tuyệt vời nhất.
Chỉ riêng tại trang chủ, chúng tôi có chèn những banner để quảng cáo các nhà cái cá cược trực tuyến hàng đầu. Đây là nguồn thu duy nhất của Ca Khia TV ở thời điểm hiện tại. Cho nên cũng mong bạn thông cảm, nếu cảm thấy bị làm phiền. Đương nhiên bạn có thể tắt những banner này bất kỳ lúc nào nếu muốn.
Những lưu ý khi xem truc tiep bong da tại Cakhia TV
Khi tiến hành xem tructiepbongda tại Cà Khịa TV, bạn cũng nên lưu ý những điều sau đây. Bởi nó giúp ích cho bạn rất nhiều, khi xem các trận đấu bóng đá mà mình thích.
- Nếu đường link bạn đang xem bong da truc tiep gặp vấn đề, bạn hãy chuyển qua link khác để tiếp tục thưởng thức trận đấu như bình thường
- Nếu như các hiện tượng giật, lag hay đứng hình xuất hiện trong quá trình trận đấu diễn ra. Lúc này bạn chỉ cần dừng trận đấu lại khoảng 1 phút. Sau đó tiếp tục xem, đảm bảo rằng các hiện tượng này sẽ biến mất ngay lập tức
- Nếu khi đang xem mà bạn bị diss ra, khả năng cao đường truyền đã bị quá tải. Lúc này bạn cũng chỉ cần vào lại được link khác là có thể tiếp tục xem được
- Để có thể xem trực tiếp các trận đấu bóng đá trên CaKhia Live một cách tốt nhất. Bạn nên lấy đường link sớm khoảng 5 phút. Như vậy trong quá trình xem, bạn sẽ rất ít khi gặp giật, lag hay là bị diss ra.
Những lý do mà bạn nên xem tructiepbongda tại Cakhia TV
Hiện tại,CaKhia Live chính là trang web mà bạn nhất định phải truy cập vào ngay lập tức. Nếu muốn xem bong da truc tiep với chất lượng cao cũng như trải nghiệm tuyệt vời. Bởi vì những lý do chính sau:
- Cà Khịa TV phát sóng bóng đá hoàn toàn miễn phí, nên bạn có thể truy cập và theo dõi trận đấu mình thích bất kỳ lúc nào
- Gần như toàn bộ các giải đấu bóng đá, từ hàng đầu trên thế giới cho đến các giải nhỏ hơn trong khu vực và trong nước đều được Cà Khịa TV phát sóng đầy đủ
- Đường link xem tructiepbongda cũng được cập nhật sớm và đa dạng cho bạn lựa chọn. Thêm vào đó, chúng tôi còn cam kết link không bao giờ dính mã độc hay là virus
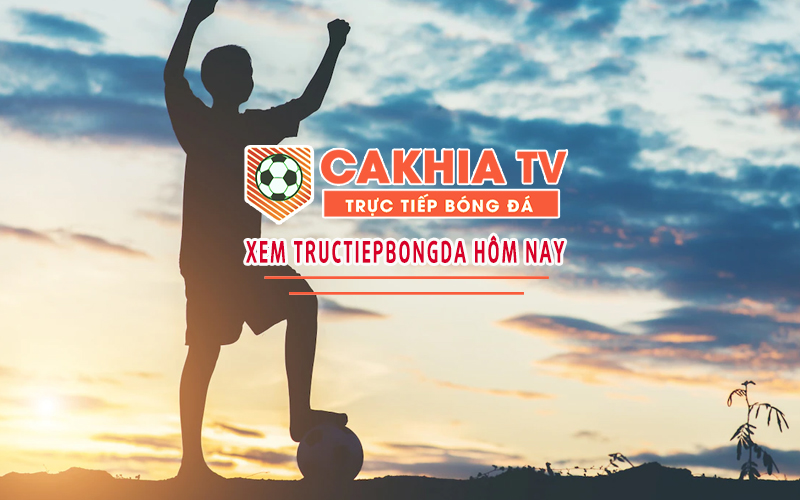
- Khi thưởng thức trận đấu mình thích tại đây, bạn sẽ cảm nhận được chất lượng cực kỳ cao. Không khác gì bạn xem trực tiếp trên TV
- Tốc độ mà trận đấu diễn ra cũng vô cùng mượt mà, ổn định và gần như không bao giờ có tình trạng giật, lag hay đứng hình xuất hiện
- Bình luận cho các trận đấu bóng đá được CaKhia T V phát sóng cũng từ những BLV có tên tuổi trong nước. Càng làm cho độ hấp dẫn và sức hút tăng lên khá nhiều
- Trải nghiệm xem của bạn cũng cực kỳ tuyệt vời, khi chúng tôi không bao giờ chèn quảng cáo khi trận đấu đã bắt đầu.
Truy cập Cakhia TV khi bạn muốn xem truc tiep bong da với chất lượng cao và trải nghiệm tuyệt vời. Đảm bảo rằng khi lựa chọn Cakhia Live, bạn sẽ không bao giờ thất vọng.
Tổng hợp một vài website xem trực tiếp bóng đá khác
Ngoài Cakhia TV là kênh bóng đá trực tiếp được đánh giá cao nhất hiện nay, chúng tôi xin liệt kê và giới thiệu thêm cho fan hâm mộ biết đến 1 số website chất lượng xếp sau Cà Khịa TV như sau:
- Xoilac TV ( Xoi Lac TV | Xoilac net | Xoilac1 | Xoilac2 | Xoilac3 | Xoilac7 | Xoilac8)
- Vaoroi TV (Vaoroi.TV | Vào Rồi TV | Vaoroi1 | Vaoroi 2 | Vaoroi5.com)
- Trực Tiếp Bóng Đá TV (Tructiepbongda TV | Tructiepbongda.TV | Truc Tiep Bong Da TV)
- Ra Khơi TV ( Rakhoi.TV | Rakhoi.link | Ra Khoi TV)
- Sao Kê TV ( Saoke.TV | Saoke.link | Sao Kê TV)
- Kèo Nhà Cái TV (Keonhacai.TV | Keonhacai TV | Keo Nha Cai TV)
- Đài VTV (VTV6 | VTV3 HD | VTV9)
- Đài VTC (VTC1 | VTC2 | VTC7)
- Đài K+ TV ( K+1 | K+ Thể Thao)
- 90Phut TV (91phut.net | 90Phut TV | 90m TV | 90P TV | 91Phut | 91Phut TV | 91Phutz.TV)
- Banthang TV ( Banthang TV | Banthang.live)
- Thuckhuya TV ( Thức Khuya TV | Thuckhuya.TV | Thuc Khuya Link | Thuc Khuya TV)
- Ngoac TV ( Ngoac.TV | Ngoác TV | Ngoạc TV | NgoacTV)
- Thevang TV ( Thẻ Vàng TV | Thevang.TV | Thevang TV | The Vang TV)
Kết luận
Trên mạng hiện tại có rất nhiều các trang web xem trực tiếp bóng đá cho mọi người lựa chọn để truy cập và xem những trận đấu bóng đá mình muốn. Tuy nhiên, đa số các website phát sóng bóng đá hiện tại vẫn đáp ứng được nhu cầu của người xem. Chính vì thế, Cakhia TV được ra đời để giúp mọi người có được một địa điểm xem bóng đá trực tuyến với chất lượng cao và trải nghiệm tốt nhất. Cakhia Liv, một địa chỉ mà bạn không nên bỏ qua, khi muốn xem bất kỳ trận đấu nào bằng hình thức trực tuyến với trải nghiệm tuyệt vời nhất.
Thông tin liên hệ với Cakhia TV
Tất cả những thắc mắc, ý kiến đóng góp sẽ đều được chúng tôi ghi nhận một cách tích cực nhất để phát triển kênh ngày càng lớn mạnh, thông tin chi tiết vui lòng liên hệ Cakhia TV dưới đây:
Đia chỉ: 158 Tô Hiệu, Hiệp Tân, Tân Phú, Hồ Chí Minh 700000.
Điện thoại: 0931528129.
Email: [email protected]
Website: https://vaccinaid.org/
Mạng Xã Hội:
- About: https://about.me/cakhiacomtv/
- Twitter: https://twitter.com/cakhiacomtv/
- Linkedin: https://www.linkedin.com/in/cakhiacomtv/
- Fanpage: https://www.facebook.com/cakhiacomtv/
- Instagram: https://instagram.com/cakhiacomtv/
- Telegram: https://t.me/s/cakhiacomtv/
Cakhia TV rất hân hạnh là nơi xem trực tiếp bóng đá tốt nhất dành cho bạn!